Datos climáticos y prácticas recomendadas para proyectar cambios en la distribución de especies
DOI:
https://doi.org/10.14522/darwiniana.2023.111.1094Palabras clave:
Datos climáticos, dispersión, ensambles, incertidumbre climática, modelos climáticos, reducción de escala, significancia de las proyeccionesResumen
El cambio climático es un proceso que impacta en todos los sistemas socio-ambientales, adquiriendo rasgos específicos en cada región. Para comprender y proyectar apropiadamente el alcance de dicho impacto, se requiere de un ejercicio conjunto entre los especialistas de las disciplinas involucradas. La comunidad científica internacional viene desarrollando diversos conjuntos de datos para el estudio de la variabilidad y del cambio climático, e ideando estrategias apropiadas para su tratamiento. Sin embargo, se observa una tendencia disciplinaria, hacia el uso de una única fuente de datos climáticos, ya sea por simplicidad o porque cumple con los requisitos de resolución o disponibilidad en el formato deseado. En este sentido, los climatólogos observan con preocupación el uso acrítico de las bases de datos climáticos. A partir de esta preocupación, surge este artículo que tiene como objetivo describir tanto los alcances como las limitaciones de las bases de datos disponibles. Además, se aborda la problemática de la incertidumbre en las proyecciones climáticas y se proporciona información sobre cómo utilizar los datos climáticos para llevar a cabo experimentos relacionados con la distribución de especies, teniendo en cuenta la incertidumbre inherente a los datos. Se destaca la importancia de realizar múltiples experimentos conducidos por N-proyecciones climáticas independientes, y de utilizar herramientas estadísticas para concluir sobre la base de una serie de posibles soluciones.
Citas
Adler, R. F. et al. 2018. The Global Precipitation Climatology Project (GPCP) monthly analysis (New Version 2.3) and a review of 2017 global precipitation. Atmosphere (Basel) 9: 138. DOI: https://doi.org/10.3390/atmos9040138
Ahrens, C. D. & R. Henson. 2018. Essentials of Meteorology: An Invitation to the Atmosphere. 8th Edition. CENGAGE Learning, Boston, USA, 550 pp.
Araújo, M. B. & M. New. 2006. Ensemble forecasting of species distributions. Trends in Ecology and Evolution 22: 42-47. DOI: https://doi.org/10.1016/j.tree.2006.09.010
Assefa, A.; A. Tibebu, A. Bihon & M. Yimana. 2021. Global ecological niche modelling of current and future distribution of peste des petits ruminants virus (PPRv) with an ensemble modelling algorithm. Transboundary and Emerging Diseases 68: 3601-3610. DOI: https://doi.org/10.1111/tbed.13967
Balmaceda Huarte, R. & M. L. Bettolli. 2022. Assessing statistical downscaling in Argentina: Daily maximum and minimum temperatures. International Journal of Climatology 42: 8423-8445.
Bedia, J.; S. Herrera & J. M. Gutiérrez. 2013. Dangers of using global bioclimatic datasets for ecological niche modeling. Limitations for future climate projections. Global and Planetary Change 107: 1-12. DOI: https://doi.org/10.1016/j.gloplacha.2013.04.005
Bergamin, R. S.; M. Gama, M. Almerão, G. S. Hofmann & P. M. Anastácio. 2022. Predicting current and future distribution of Hovenia dulcis Thunb. (Rhamnaceae) worldwide. Biological Invasions 24: 2229-2243. DOI: https://doi.org/10.1007/s10530-022-02771-0
Bettolli, M. L. et al. 2021. The CORDEX Flagship Pilot Study in southeastern South America: a comparative study of statistical and dynamical downscaling models in simulating daily extreme precipitation events. Climate Dynamics 56: 1589-1608. DOI: https://doi.org/10.1007/s00382-020-05549-z
Booth, T. H. 2022. Checking bioclimatic variables that combine temperature and precipitation data before their use in species distribution models. Austral Ecology 47: 1506-1514. DOI: https://doi.org/10.1111/aec.13234
Boulanger, J.-P.; A. F. Carril & E. Sanchez. 2016. CLARIS-La Plata Basin: regional hydroclimate variability, uncertainties and climate change scenarios. Climate Research 68: 93-94. DOI: https://doi.org/10.3354/cr01392
Carril, A. F. et al. 2012. Performance of a multi-RCM ensemble for South Eastern South America. Climate Dynamics 39: 2747-2768. DOI: https://doi.org/10.1007/s00382-012-1573-z
Casanueva, A.; S. Herrera, J. Fernández & J. M. Gutiérrez. 2016. Towards a fair comparison of statistical and dynamical downscaling in the framework of the EURO-CORDEX initiative. Climatic Change 137: 411-426. DOI: https://doi.org/10.1007/s10584-016-1683-4
Chen, D. et al. 2021. Framing, Context, and Methods. Chapter 1 in “Climate Change 2021: The Physical Science Basis”. Contribution of Working Group I to the Sixth Assessment Report of the Intergovernmental Panel on Climate Change, Cambridge University Press, Cambridge, United Kingdom and New York, NY, USA, 147-286.
Chen, M.; W. Shi, P. Xie, V. B. S. Silva, V. E. Kousky, R. W. Higgins & J. E. Janowiak. 2008. Assessing objective techniques for gauge-based analyses of global daily precipitation. Journal of Geophysical Research Atmospheres 113: 1-13. DOI: https://doi.org/10.1029/2007JD009132
Christensen, J. H.; B. Hewitson, A. Busuioc, A. Chen, X. Gao, I. Held, R. Jones, R. K. Kolli, W. T. Kwon, R. Laprise, V. Magana Rueda, L. Mearns, C. G. Menéndez, J. Raisanen, A. Rinke, A. Sarr & P. Whetton. 2007. Regional Climate Projections. En: S. Solomon, D. Qin, M. Manning, Z. Chen, M. Marquis, K.B. Averyt, M. Tignor & H. L. Miller (Eds.) Climate Change 2007: The Physical Science Basis. Contribution of Working Group I to the Fourth Assessment Report of the Intergovernmental Panel on Climate Change, Cambridge University Press, Cambridge, New York.
Deblauwe, V.; V. Droissart, R. Bose, B. Sonké, A. Blach-Overgaard, J.-C. Svenning, J. J. Wieringa, B. R. Ramesh, T. Stévart & T. L. P. Couvreur. 2016. Remotely sensed temperature and precipitation data improve species distribution modelling in the tropics. Global Ecology and Biogeography 25: 443-454. DOI: https://doi.org/10.1111/geb.12426
Dee, D. P. et al. 2011. The ERA-Interim reanalysis: Configuration and performance of the data assimilation system. Quarterly Journal of the Royal Meteorological Society 137: 553-597. DOI: https://doi.org/10.1002/qj.828
Duchon, J. 1977. Splines minimizing rotation invariant seminorms in sobolev spaces, constructive theory of functions of several variables. Lecture Notes in Mathematics 571: 85-100. DOI: https://doi.org/10.1007/BFb0086566
Edenhofer, O. & K. Seyboth. 2013. Intergovernmental Panel on Climate Change (IPCC). Encyclopedia of Energy, Natural Resource, and Environmental Economics, J. F. Shogren, Ed., Elsevier Inc., 48-56. DOI: https://doi.org/10.1016/B978-0-12-375067-9.00128-5
Escobar, L. E.; A. Lira-Noriega, G. Medina-Vogel & A. Townsend Peterson. 2014. Potential for spread of the white-nose fungus (Pseudogymnoascus destructans) in the Americas: Use of Maxent and NicheA to assure strict model transference. Geospatial Health 9: 221-229. DOI: https://doi.org/10.4081/gh.2014.19
Evans, M. E. K.; C. Merow, S. Record, S. M. McMahon & B. J. Enquist. 2016. Towards Process-based Range Modeling of Many Species. Trends in Ecology and Evolution 31: 860-871. DOI: https://doi.org/10.1016/j.tree.2016.08.005
Eyring, V.; S. Bony, G. A. Meehl, C. A. Senior, B. Stevens, R. J. Stouffer & K. E. Taylor. 2016. Overview of the Coupled Model Intercomparison Project Phase 6 (CMIP6) experimental design and organization. Geoscientific Model Development 9: 1937-1958. DOI: https://doi.org/10.5194/gmd-9-1937-2016
Falco, M. 2019: Modelado climático en Sudamérica: el valor agregado por los Modelos Climáticos Regionales. PhD. Thesis, University of Buenos Aires, Argentina, 175 pp.
Falco, M.; A. F. Carril, L. Z. X. Li, C. Cabrelli & C. G. Menéndez. 2019a. The potential added value of Regional Climate Models in South America using a multiresolution approach. Climate Dynamics 54: 1553-1569. DOI: https://doi.org/10.1007/s00382-019-05073-9
Falco, M.; A. F. Carril, C. G. Menéndez, P. G. Zaninelli & L. Z. X. Li. 2019b. Assessment of CORDEX simulations over South America: added value on seasonal climatology and resolution considerations. Climate Dynamics 52: 4771-1786. DOI: https://doi.org/10.1007/s00382-018-4412-z
Falloon, P.; A. Challinor, S. Dessai, L. Hoang, J. Johnson & A. K. Koehler. 2014. Ensembles and uncertainty in climate change impacts. Frontiers in Environmental Science 2: 1-7. DOI: https://doi.org/10.3389/fenvs.2014.00033
Fergnani, P. N. 2022. R como un SIG: Extracción de datos climáticos de WorldClim. Ecología Austral 32: 45-54. DOI: https://doi.org/10.25260/ea.22.32.1.0.1119
Fick, S. E. & R. J. Hijmans. 2017. WorldClim 2: new 1-km spatial resolution climate surfaces for global land areas. International Journal of Climatology 37: 4302-4315. DOI: https://doi.org/10.1002/joc.5086
Flato, G. et al. 2013. Evaluation of Climate Models. Climate Change 2013: The Physical Science Basis. Contribution of Working Group I to the Fifth Assessment Report of the Intergovernmental Panel on Climate Change. En: T. F. Stocker et al. (Eds.), Cambridge University Press, Cambridge, United Kingdom and New York, NY, USA.
Flombaum, P.; L. Yahdjian & O. E. Sala. 2017. Global-change drivers of ecosystem functioning modulated by natural variability and saturating responses. Global Change Biology 23: 503-511. DOI: https://doi.org/10.1111/gcb.13441
García Skabar, Y. & M. Nicolini. 2014. Impact of enriched analyses on regional numerical forecasts over southeastern South America during SALLJEX. Revista Brasileira de Meteorologia 29: 315-330. DOI: https://doi.org/10.1590/0102-778620100248
Giorgi, F. 2006. Regional climate modeling: Status and perspectives. Journal de Physique IV 139: 101-118. DOI: https://doi.org/10.1051/jp4:2006139008
Giorgi, F. 2010. Uncertainties in climate change projections, from the global to the regional scale. EPJ Web Conference 9: 115-129. DOI: https://doi.org/10.1051/epjconf/201009009
Giorgi, F. 2019. Thirty Years of Regional Climate Modeling: Where Are We and Where Are We Going next? Journal of Geophysical Research: Atmospheres 124: 5696-5723. DOI: https://doi.org/10.1029/2018JD030094
Goosse, H. 2015. Climate System Dynamics and Modelling. Cambridge University Press, 273 pp.
Guisan, A.; A. Lehmann, S. Ferrier, M. Austin, J. M. C. Overton, R. Aspinall & T. Hastie. 2006. Making better biogeographical predictions of species’ distributions. Journal of Applied Ecology 43: 386-392. DOI: https://doi.org/10.1111/j.1365-2664.2006.01164.x
Guisan, A.; W. Thuiller & N. E. Zimmermann. 2017. Habitat Suitability and Distribution Models: with Applications in R (Ecology, Biodiversity and Conservation). Cambridge: Cambridge University Press. DOI: https://doi.org/10.1017/9781139028271
Gutiérrez, J. M.; D. San-Martín, S. Brands, R. Manzanas & S. Herrera. 2013. Reassessing statistical downscaling techniques for their robust application under climate change conditions. Journal of Climate 26: 171-188. DOI: https://doi.org/10.1175/JCLI-D-11-00687.1
Gutowski, W. J.; F. Giorgi, B. Timbal, A. Frigon, D. Jacob, H.-S. Kang, K. Raghavan, B. Lee, C. Lennard, G. Nikulin, E. O’Rourke, M. Rixen, S. Solman, T. Stephenson & F. Tangang. 2016. WCRP COordinated Regional Downscaling EXperiment (CORDEX): a diagnostic MIP for CMIP6. Geoscientific Model Development 9: 4087-4095. DOI: https://doi.org/10.5194/gmd-9-4087-2016
Halsnæs, K. & P. S. Kaspersen. 2018. Decomposing the cascade of uncertainty in risk assessments for urban flooding reflecting critical decision-making issues. Climatic Change 151: 491-506. DOI: https://doi.org/10.1007/s10584-018-2323-y
Harms, D. E.; S. Raman & R. V. Madala. 1992. An examination of four-dimensional data-assimilation techniques for numerical weather prediction. Bulletin of the American Meteorological Society 73: 425-440. DOI: https://doi.org/10.1175/1520-0477(1992)073<0425:AEOFDD>2.0.CO;2
Harris, I.; T. J. Osborn, P. Jones & D. Lister. 2020. Version 4 of the CRU TS monthly high-resolution gridded multivariate climate dataset. Scientific Data 7: 1-18. DOI: https://doi.org/10.1038/s41597-020-0453-3
Hattermann, F. F. et al. 2018. Sources of uncertainty in hydrological climate impact assessment: A cross-scale study. Environmental Research Letters 13: 015006. DOI: https://doi.org/10.1088/1748-9326/aa9938
Hawkins, E. & R. Sutton. 2009. The potential to narrow uncertainty in regional climate predictions. Bulletin of the American Meteorological Society 90: 1095-1107. DOI: https://doi.org/10.1175/2009BAMS2607.1
Hawkins, E. & R. Sutton. 2011. The potential to narrow uncertainty in projections of regional precipitation change. Climate Dynamics 37: 407-418. DOI: https://doi.org/10.1007/s00382-010-0810-6
Hay, L. E.; R. L. Wilby & G. H. Leavesley. 2000. A comparison of delta change and downscaled GCM scenarios for three mountainous basins in the United States. Journal of the American Water Resources Association 36: 387-397. DOI: https://doi.org/10.1111/j.1752-1688.2000.tb04276.x
Hersbach, H. et al. 2020. The ERA5 global reanalysis. Quarterly Journal of the Royal Meteorological Society 146: 1999-2049. DOI: https://doi.org/10.1002/qj.3803
Hijmans, R. J.; S. E. Cameron, J. L. Parra, P. G. Jones & A. Jarvis. 2005. Very high resolution interpolated climate surfaces for global land areas. International Journal of Climatology 25: 1965-1978. DOI: https://doi.org/10.1002/joc.1276
Hurrell, J. W. 1995. Decadal trends in the North Atlantic Oscillation: Regional temperatures and precipitation. Science 269: 676-679.
Karger, D. N. et al. 2017. Climatologies at high resolution for the earth’s land surface areas. Scientific Data 4: 1-20. DOI: https://doi.org/10.1038/sdata.2017.122
Karoly, D. J. 1989. Southern Hemisphere circulation features associated with El Niño-Southern Oscillation events. Journal of Climate 2: 1239-1252. DOI: https://doi.org/10.1175/1520-0442(1989)002<1239
Katz, R. W.; P. F. Craigmile, P. Guttorp, M. Haran, B. Sansó & M. L. Stein. 2013. Uncertainty analysis in climate change assessments. Nature Climate Change 3: 769-771. DOI: https://doi.org/10.1038/nclimate1980
Kawamiya, M.; T. Hajima, K. Tachiiri, S. Watanabe & T. Yokohata. 2020. Two decades of Earth system modeling with an emphasis on Model for Interdisciplinary Research on Climate (MIROC). Progress in Earth and Planetary Science 7: 64. DOI: https://doi.org/10.1186/s40645-020-00369-5
Kendon, E. J.; D. P. Rowell, R. G. Jones & E. Buonomo. 2008. Robustness of future changes in local precipitation extremes. Journal of Climate 21: 4280-4297. DOI: https://doi.org/10.1175/2008JCLI2082.1
Kidson, J. W. 1999. Principal Modes of Southern Hemisphere Low-Frequency Variability Obtained from NCEP-NCAR Reanalyses. Journal of Climate 2808-2830.
Kobayashi, S. et al. 2015. The JRA-55 Reanalysis: General Specifications and Basic Characteristics. Journal of the Meteorological Society of Japan. Ser. II 93: 5-48. DOI: https://doi.org/10.2151/jmsj.2015-001
Lange, S. 2019. Trend-preserving bias adjustment and statistical downscaling with ISIMIP3BASD (v1.0). Geoscientific Model Development 12: 3055-3070. DOI: https://doi.org/10.5194/gmd-12-3055-2019
Lee, J.-Y. et al. 2021. Future global climate: scenario-based projections and near-term information. Chapter 4 in “Climate Change 2021: The Physical Science Basis”. Contribution of Working Group I to the Sixth Assessment Report of the Intergovernmental Panel on Climate Change, Cambridge University Press, Cambridge, United Kingdom and New York, USA, 553-672.
Maraun, D. et al. 2010. Precipitation downscaling under climate change: Recent developments to bridge the gap between dynamical models and the end user. Reviews of Geophysics 48: 1-34. DOI: https://doi.org/10.1029/2009RG000314
Maraun, D. et al. 2017. Towards process-informed bias correction of climate change simulations. Nature Climate Change 7: 764-773. DOI: https://doi.org/10.1038/nclimate3418
Navarro-Racines, C.; J. Tarapues, P. Thornton, A. Jarvis & J. Ramirez-Villegas. 2020. High-resolution and bias-corrected CMIP5 projections for climate change impact assessments. Scientific Data 7: 1-14. DOI: https://doi.org/10.1038/s41597-019-0343-8
O’Neill, B. C. et al. 2016. The Scenario Model Intercomparison Project (ScenarioMIP) for CMIP6. Geoscientific Model Development 9: 3461-3482. DOI: https://doi.org/10.5194/gmd-9-3461-2016
Organización Meteorológica Mundial. 2021. Estado del Sistema Mundial de Observación del Clima.
Pacheco, S.; L. R. Malizia & L. Cayuela. 2010. Effects of climate change on subtropical forests of South America. Tropical Conservation Science 3: 423-437. DOI: https://doi.org/10.1177/194008291000300407
Quesada-Chacón, D.; K. Barfus & C. Bernhofer. 2021. Climate change projections and extremes for Costa Rica using tailored predictors from CORDEX model output through statistical downscaling with artificial neural networks. International Journal of Climatology 41: 211-232. DOI: https://doi.org/10.1002/joc.6616
Riahi, K. et al. 2017. The Shared Socioeconomic Pathways and their energy, land use, and greenhouse gas emissions implications: An overview. Global Environmental Change 42: 153-168. DOI: https://doi.org/10.1016/j.gloenvcha.2016.05.009
Saha, S. et al. 2013. The NCEP Climate Forecast System Version 2. Journal of Climate 27: 2185-2208. DOI: https://doi.org/10.1175/jcli-d-12-00823.1
Salio, P.; M. P. Hobouchian, Y. García Skabar & D. Vila. 2015. Evaluation of high-resolution satellite precipitation estimates over southern South America using a dense rain gauge network. Atmospheric Research 163: 146-161. DOI: https://doi.org/10.1016/j.atmosres.2014.11.017
Silva, D. P.; S. Varela, A. Nemesio & P. De Marco. 2015. Adding Biotic Interactions into Paleodistribution Models: A Host-Cleptoparasite Complex of Neotropical Orchid Bees. PLoS One 10(6): e0129890. DOI: https://doi.org/10.1371/journal.pone.0129890
Solman, S. A. et al. 2013. Evaluation of an ensemble of regional climate model simulations over South America driven by the ERA-Interim reanalysis: Model performance and uncertainties. Climate Dynamics 41: 1139-1157. DOI: https://doi.org/10.1007/s00382-013-1667-2
Song, X.; D. Y. Wang, F. Li & X. D. Zeng. 2021. Evaluating the performance of CMIP6 Earth system models in simulating global vegetation structure and distribution. Advances in Climate Change Research 12: 584-595. DOI: https://doi.org/10.1016/j.accre.2021.06.008
Soria-Auza, R. W.; M. Kessler, K. Bach, P. M. Barajas-Barbosa, M. Lehnert, S. K. Herzog & J. Böhner. 2010. Impact of the quality of climate models for modelling species occurrences in countries with poor climatic documentation: a case study from Bolivia. Ecological Modelling 221: 1221-1229. DOI: https://doi.org/10.1016/j.ecolmodel.2010.01.004
Stocker, T. F. & D. Qin. 2014. Climate Change 2013. The Physical Science Basis. Frequently Asked Questions. IPCC, WG1, 78.
Tapiador, F. J.; A. Navarro, R. Moreno, J. L. Sánchez & E. García-Ortega. 2020. Regional climate models: 30 years of dynamical downscaling. Atmospheric Research 235: 104785. DOI: https://doi.org/10.1016/j.atmosres.2019.104785
Tovar, C. et al. 2022. Understanding climate change impacts on biome and plant distributions in the Andes: Challenges and opportunities. Journal of Biogeography 49: 1420-1442. DOI: https://doi.org/10.1111/jbi.14389
Trenberth, K. E. 1992. Climate System Modeling. K. E. Trenberth, Ed. Cambridge University Press, NY, USA, 788 pp.
Urrutia, R. & M. Vuille. 2009. Climate change projections for the tropical Andes using a regional climate model: Temperature and precipitation simulations for the end of the 21st century. Journal of Geophysical Research 114: D2.
Varela, S.; J. M. Lobo & J. Hortal. 2011. Using species distribution models in paleobiogeography: A matter of data, predictors and concepts. Palaeogeography, Palaeoclimatology, Palaeoecology 310: 451-463. DOI: https://doi.org/10.1016/j.palaeo.2011.07.021
Varela, S.; L. C. Terribile, G. de Oliveira, J. A. F. Diniz-Filho, J. González-Hernández &M. S. Lima-Ribeiro. 2015. ecoClimate vs. Worldclim: variables climáticas SIG para trabajar en biogeografía. Ecosistemas 24: 88-92.
Whittaker, R. H. 1975. Community Structure and Composition. Chapter 3 in Communities and Ecosystems., MacMillan Publishing Co., New York, 60-65.
Wilby, R. L. & T. M. L. Wigley. 1997. Downscaling general circulation model output: a review of methods and limitations. Progress in Physical Geography 21: 530-548.
Wilby, R. L. & S. Dessai. 2010. Robust adaptation to climate change. Weather 65: 180-185. DOI: https://doi.org/10.1002/wea.543
Wilby, R. L.; T. M. L. Wigley, D. Conway, P. D. Jones, B. C. Hewitson, J. Main & D. S. Wilks. 1998. Statistical downscaling of general circulation model output: A comparison of methods. Water Resources Research 34: 2995-3008. DOI: https://doi.org/10.1029/98WR02577
Xie, P.; A. Yatagai, M. Chen, T. Hayasaka, Y. Fukushima, C. Liu & S. Yang. 2007. A gauge-based analysis of daily precipitation over East Asia. Journal of Hydrometeorology 8: 607-626. DOI: https://doi.org/10.1175/JHM583.1
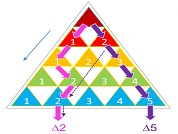
Descargas
Publicado
Cómo citar
Número
Sección
Licencia
A partir de 2012, esta obra está licenciada bajo una Licencia Creative Commons Atribución-NoComercial 2.5 Argentina .
Cualquier obra derivada deberá estar previamente autorizada con nota escrita de los editores.